0%
URL
TL;DR
- 本文在
GShard
的基础上,提出了一种新的混合专家语言模型 DeepSeekMoE
,通过 孤立共享专家 和 细分专家 的方式,提高了模型性能并降低了计算复杂度。
- 替换的也是
Transformer
中的 FFN
层。
Architecture
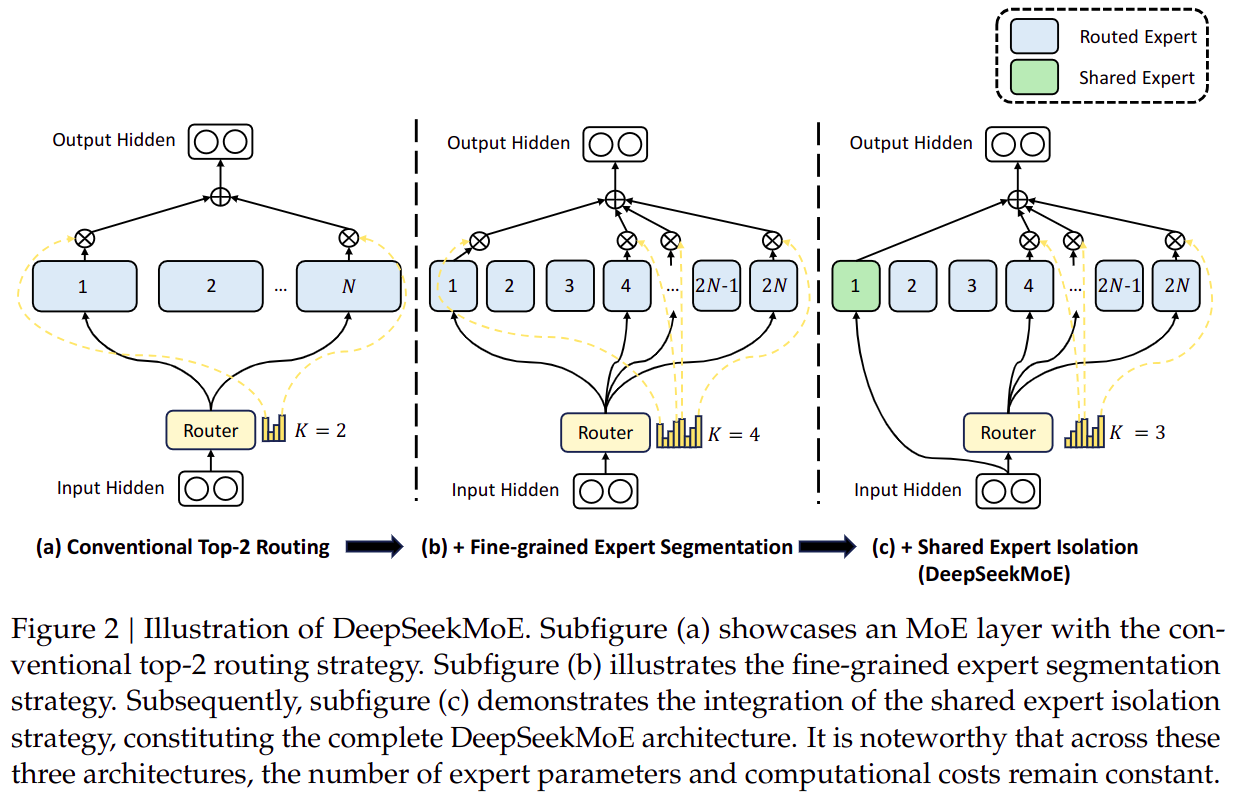
传统 MoE 模型(例如 GShard
)
- 传统
MoE
如图 a
所示,核心思想是将 Transformer
的 FFN
替换为 MoE
,每个 token
通过 Gate
机制选择不同的 Expert
来处理。
- 用公式表示为:
- htl=∑i=1N(gi,tFFNi(utl))+utl
- gi,t={si,t,0,si,t∈TopK(sj,t∣1≤j≤N,K),otherwise,
- si,t=Softmax(utlTeil)
- 其中:
l
表示第 l
层
N
表示 Expert
的数量
K
表示每个 token
保留的 Expert
数量
细粒度 MoE 模型
- 如图
b
所示,和传统 MoE
的区别是将专家切分的更小,专家数量更多,也可以理解为传统 MoE
中的 Expert
也是由多个 Sub-Expert
组成。
- 用公式表示为:
- htl=∑i=1mN(gi,tFFNi(utl))+utl
- gi,t={si,t,0,si,t∈TopK(sj,t∣1≤j≤mN,mK),otherwise,
- si,t=Softmax(utlTeil)
- 其中:
l
表示第 l
层
N
表示 Expert
的数量
m
表示每个 Expert
中包含的 Sub-Expert
的数量
K
表示每个 token
保留的 Expert
数量
细粒度 MoE + 孤立共享专家
- 如图
c
所示,在细粒度 MoE
的基础上,引入了 Isolated Shared Expert
,这种专家不参与 Gate
选择,而是在所有 token
之间共享。
- 用公式表示为:
- htl=∑i=1KsFFNi(utl)+∑i=Ks+1mN(gi,tFFNi(utl))+utl
- gi,t={si,t,0,si,t∈TopK(sj,t∣Ks+1≤j≤mN,mK−Ks),otherwise,
- si,t=Softmax(utlTeil)
- 其中:
l
表示第 l
层
N
表示 Expert
的数量
m
表示每个 Expert
中包含的 Sub-Expert
的数量
K
表示每个 token
保留的 Expert
数量
- Ks 表示
Isolated Shared Expert
的数量
Thoughts
- 这篇论文名字起的有点大《迈向终极专家专业化的MoE语言模型》,但是实际上只是在
GShard
的基础上做了一些小的改进。